Using Machine Learning to Classify Treatment Approaches for Infants with Bronchopulmonary Dysplasia
Using Machine Learning to Classify Treatment Approaches for Infants with Bronchopulmonary Dysplasia https://pediatricsnationwide.org/wp-content/uploads/2023/08/091021BS0157-1024x683.jpg 1024 683 Pam Georgiana Pam Georgiana https://pediatricsnationwide.org/wp-content/uploads/2023/07/May-2023.jpg- August 30, 2023
- Pam Georgiana
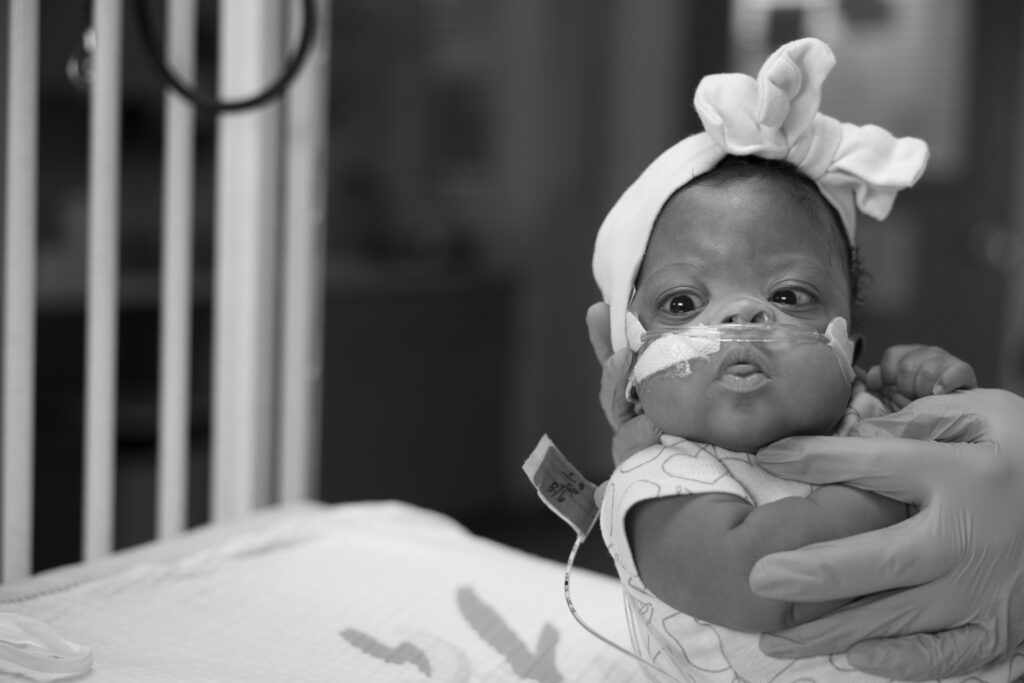
We don’t yet know the best way to help infants with severe bronchopulmonary dysplasia (BPD) breathe using ventilators. Currently, doctors across the country and around the world use a variety of different approaches. These variations depend on the severity of a patient’s medical condition, the ventilator settings used, and location-specific standards.
Matthew Kielt, MD, a neonatologist at Nationwide Children’s Hospital and an assistant professor of Clinical Pediatrics at The Ohio State University College of Medicine, and others wanted to analyze recent treatment data using machine learning techniques to uncover which ventilator approaches are most used together when treating babies with severe BPD.[1] This could help medical professionals understand their care better, make clinical trials more effective, and contribute to developing more personalized and effective ventilation protocols in the future.
Dr. Kielt’s team studied 78 patients with severe BPD from 14 different hospitals. They organized the group based on these four ventilator settings:
- Mean airway pressure (MAP)
- Positive end expiratory pressure (PEEP)
- Set respiratory rate
- Inspiratory time (Ti)
The team used Ward’s hierarchical clustering analysis (HCA) to group data points based on their similarities. This unsupervised clustering analysis method uses machine learning to organize data points into groups without being explicitly told how to do so. Unlike supervised methods, where a computer uses labeled examples to learn from, unsupervised clustering doesn’t have predefined categories. Instead, it uses mathematical algorithms to group the data in a way that reveals inherent structures or relationships within the dataset. This creates groups of similar data points at different levels of the hierarchy. It’s a technique often used in data analysis to uncover patterns and relationships within complex, heterogeneous datasets.
Dr. Kielt and team found three discrete approaches to mechanical ventilation where the median PEEP, MAP, respiratory rate, Ti, and peak inspiratory pressure (PIP) differed significantly between clusters. Most often, clinicians used two different approaches for infants with severe disease features, and a third approach with infants who likely had a milder form of disease. Further study is needed to determine whether these approaches are best associated with specific types of BPD and if they affect breathing outcomes differently.
“It’s too soon to say any of these approaches should become the standard. This study was looking at a snapshot in time. We need to look at longitudinal data to determine both short and long-term outcomes of infants treated with these clustered approaches,” Dr. Kielt says. “We also need to study how these approaches can be incorporated into clinical weaning protocols for ventilator-dependent infants with BPD.”
Aside from the potential innovations in care this study uncovers, it is also clear to Dr. Kielt that there is value in using machine learning for further studies.
“Machine learning can robustly classify data sets in novel ways that completely remove human bias. So often, medical professionals get stuck on specific yet under-tested processes or treatments. This can limit the possibilities of new and innovative ideas,” says Dr. Kielt.
The next steps are already underway, and Dr. Kielt is looking forward to a clinical trial soon.
“We are headed in a very exciting direction. This study is an important first step in developing more robust clinical trials and quality improvement initiatives to better define ventilator strategies and respiratory outcomes for these very sick infants,” Dr. Kielt says.
Reference:
[1] Kielt MJ, Hatch LD 3rd, Levin JC, et al. Classifying multicenter approaches to invasive mechanical ventilation for infants with bronchopulmonary dysplasia using hierarchical clustering analysis. Pediatric Pulmonology. 2023;58(8):2323-2332. doi:10.1002/ppul.26488
Image credit: Nationwide Children’s
About the author
Pam Georgiana is a brand marketing professional and writer located in Bexley, Ohio. She believes that words bind us together as humans and that the best stories remind us of our humanity. She specialized in telling engaging stories for healthcare, B2B services, and nonprofits using classic storytelling techniques. Pam has earned an MBA in Marketing from Capital University in Columbus, Ohio.
-
Pam Georgianahttps://pediatricsnationwide.org/author/pam-georgiana/
-
Pam Georgianahttps://pediatricsnationwide.org/author/pam-georgiana/
-
Pam Georgianahttps://pediatricsnationwide.org/author/pam-georgiana/August 30, 2023
-
Pam Georgianahttps://pediatricsnationwide.org/author/pam-georgiana/